In the world of data management and analytics, raw distribution plays a crucial role in shaping how businesses and organizations interpret information. This term refers to the initial form of data collected directly from its source without any alterations or modifications. Understanding raw distribution is vital for anyone involved in data science, statistics, or business intelligence. By exploring this concept, you can uncover its importance and relevance in modern data-driven decision-making processes.
Raw data is often considered messy and unstructured, but its untouched nature provides a unique perspective that processed data cannot offer. Many professionals overlook the value of raw distribution, focusing instead on cleaned or transformed datasets. However, the initial state of data can reveal patterns and insights that are lost during processing. This article will delve into the significance of raw distribution and its impact on various industries.
Whether you're a data scientist, analyst, or simply someone curious about how data shapes our world, understanding raw distribution is essential. This comprehensive guide will explore its definition, applications, challenges, and best practices. By the end, you'll have a deeper appreciation for the importance of raw data and how it can enhance your analytical capabilities.
Read also:How To Build A Successful Online Business In 2023
What is Raw Distribution?
Raw distribution refers to the initial state of data collected directly from its source. Unlike processed or cleaned data, raw distribution retains all its original characteristics, including errors, inconsistencies, and missing values. This untouched form of data provides a complete picture of the information being analyzed, allowing analysts to identify patterns and insights that might be lost during processing.
In technical terms, raw distribution represents the frequency of occurrence of each value in a dataset without any aggregation or transformation. This allows analysts to observe the natural variability and distribution of data points, which is crucial for accurate analysis and interpretation.
Characteristics of Raw Distribution
- Unprocessed: Raw distribution remains in its original form without any modifications.
- Complete: It includes all data points, even those with errors or inconsistencies.
- Varied: The data may exhibit a wide range of values and patterns.
- Untouched: No transformations or aggregations have been applied.
Why is Raw Distribution Important?
Raw distribution holds significant importance in data analysis and decision-making processes. Its untouched nature allows analysts to explore data in its purest form, revealing insights that might be obscured by processing. By understanding raw distribution, professionals can make more informed decisions and develop strategies based on accurate information.
For example, in the healthcare industry, raw distribution can help identify patterns in patient data that might indicate potential health risks. In finance, it can reveal anomalies in transaction data that could signal fraudulent activities. Across various sectors, raw distribution serves as a foundational element for effective data analysis.
Applications of Raw Distribution
Data Science
Data scientists rely heavily on raw distribution to develop models and algorithms. By analyzing raw data, they can identify correlations, trends, and anomalies that inform predictive analytics and machine learning processes. This approach ensures that models are built on a solid foundation of accurate and comprehensive data.
Business Intelligence
Businesses use raw distribution to gain insights into customer behavior, market trends, and operational performance. By examining unprocessed data, organizations can uncover opportunities for improvement and innovation. This capability is particularly valuable in competitive industries where data-driven decisions can provide a significant advantage.
Read also:Losertown Calorie Calculator Your Ultimate Guide To Effective Calorie Management
Healthcare
In healthcare, raw distribution plays a critical role in patient monitoring and treatment planning. By analyzing raw data from medical devices and electronic health records, healthcare providers can detect early warning signs of health issues and implement timely interventions. This approach enhances patient outcomes and improves overall healthcare delivery.
Challenges in Working with Raw Distribution
While raw distribution offers numerous benefits, it also presents several challenges. The unprocessed nature of the data can make it difficult to work with, requiring specialized tools and techniques to manage effectively. Additionally, the presence of errors and inconsistencies can complicate analysis and interpretation.
Data Cleaning
Data cleaning is a crucial step in working with raw distribution. This process involves identifying and correcting errors, removing duplicates, and handling missing values. While time-consuming, data cleaning ensures that the analysis is based on accurate and reliable information.
Data Transformation
Transforming raw data into a usable format is another challenge. This may involve normalization, aggregation, or other techniques to make the data more manageable. Effective data transformation requires a deep understanding of both the data and the analysis objectives.
Best Practices for Handling Raw Distribution
To maximize the value of raw distribution, it's essential to follow best practices in data management and analysis. These practices ensure that the data remains accurate, reliable, and useful throughout the analysis process.
- Use robust data management tools to organize and store raw data effectively.
- Implement thorough data cleaning processes to address errors and inconsistencies.
- Apply appropriate data transformation techniques to prepare data for analysis.
- Document all steps and transformations to maintain transparency and reproducibility.
Tools and Technologies for Raw Distribution
Several tools and technologies are available to assist with raw distribution analysis. These include:
Data Management Platforms
Platforms like Apache Hadoop and Apache Spark provide scalable solutions for managing large volumes of raw data. These tools enable efficient processing and analysis, making them ideal for big data applications.
Statistical Software
Software such as R and Python offer powerful libraries and frameworks for analyzing raw distribution. These tools allow analysts to explore data, identify patterns, and develop predictive models with ease.
Real-World Examples of Raw Distribution
To better understand the practical applications of raw distribution, consider the following examples:
Finance
In the financial sector, raw distribution helps detect fraudulent transactions by identifying unusual patterns in transaction data. This capability is essential for maintaining the integrity of financial systems and protecting consumers.
Retail
Retailers use raw distribution to analyze customer purchase behavior and optimize inventory management. By examining unprocessed sales data, they can identify trends and make informed decisions about product offerings and promotions.
Future Trends in Raw Distribution
The field of raw distribution is evolving rapidly, driven by advancements in technology and increasing data volumes. Emerging trends include:
- Edge computing for real-time data processing.
- Artificial intelligence and machine learning for advanced analytics.
- Blockchain technology for secure data management.
These developments promise to enhance the capabilities of raw distribution, enabling more accurate and insightful analyses across various industries.
Conclusion
Raw distribution is a fundamental aspect of data analysis that offers significant value in understanding and interpreting information. By exploring its definition, applications, challenges, and best practices, this article has provided a comprehensive overview of its importance in modern data-driven decision-making processes.
We encourage you to apply the knowledge gained from this article to your own data analysis projects. Share your thoughts and experiences in the comments below, and don't forget to explore other articles on our site for more insights into the world of data science and analytics.
Table of Contents
- What is Raw Distribution?
- Why is Raw Distribution Important?
- Applications of Raw Distribution
- Challenges in Working with Raw Distribution
- Best Practices for Handling Raw Distribution
- Tools and Technologies for Raw Distribution
- Real-World Examples of Raw Distribution
- Future Trends in Raw Distribution
- Conclusion

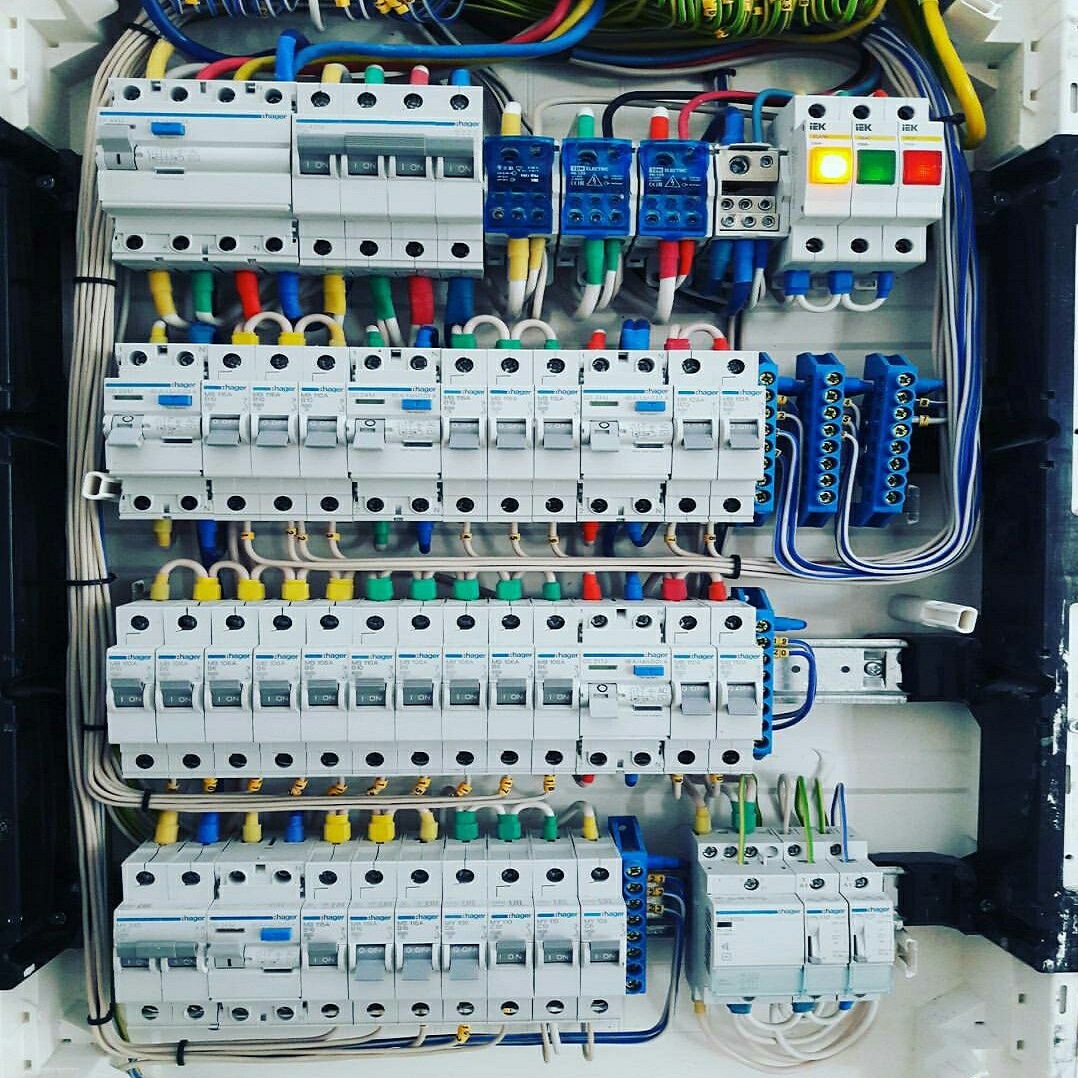