Stateful large language model serving with Pensieve has become a pivotal advancement in artificial intelligence deployment, offering solutions that cater to real-time data processing and user interaction. As the demand for AI-driven applications grows, so does the need for scalable and efficient serving systems. Pensieve's innovative approach ensures that models can maintain stateful interactions while delivering high performance and accuracy. This breakthrough is reshaping how businesses and developers handle complex AI tasks.
Artificial intelligence has evolved significantly over the years, with large language models (LLMs) playing a central role in driving innovation. These models have the potential to revolutionize industries ranging from healthcare to finance. However, deploying these models efficiently and maintaining their stateful interactions remains a challenge. This is where Pensieve steps in, providing a robust framework for stateful large language model serving.
In this article, we will delve into the intricacies of Pensieve and its role in stateful LLM serving. We'll explore the technical aspects, benefits, challenges, and future prospects of this technology. Whether you're a developer, researcher, or business professional, understanding Pensieve's capabilities can help you harness the full potential of AI in your projects.
Read also:Irelia Atwood The Rising Star Of Digital Influence And Creativity
Below is a table of contents that will guide you through the article:
- Overview of Stateful Large Language Models
- Introduction to Pensieve
- Pensieve's Architecture
- Benefits of Using Pensieve
- Challenges in Stateful Model Serving
- Use Cases of Pensieve
- Comparison with Other Systems
- Optimizing Pensieve Performance
- Future of Stateful LLM Serving
- Conclusion
Overview of Stateful Large Language Models
What Are Stateful Models?
Stateful large language models are AI models capable of remembering and utilizing past interactions to enhance future responses. Unlike stateless models, which treat each input independently, stateful models maintain a memory of previous inputs and outputs. This feature is particularly useful in applications requiring context-aware interactions, such as chatbots and virtual assistants.
Key characteristics of stateful models include:
- Memory retention across sessions
- Context-aware processing
- Adaptability to user preferences
Importance in AI Applications
The significance of stateful models in AI applications cannot be overstated. They enable more natural and engaging user interactions by providing responses that are not only accurate but also contextually relevant. For instance, in customer service chatbots, stateful models can remember a user's previous queries, ensuring a seamless and personalized experience.
According to a report by Gartner, businesses leveraging stateful AI models can achieve a 30% increase in customer satisfaction rates. This statistic underscores the importance of adopting such models for enhancing user engagement.
Introduction to Pensieve
What Is Pensieve?
Pensieve is an advanced framework designed for stateful large language model serving. Developed to address the challenges of deploying stateful models at scale, Pensieve offers a comprehensive solution for managing and optimizing these models. Its architecture is built to handle the complexities of real-time data processing and interaction.
Read also:Izzy Green Bj Exploring The Rise And Legacy Of A Music Sensation
Key features of Pensieve include:
- Scalable deployment capabilities
- Efficient memory management
- Integration with existing AI pipelines
Pensieve's Role in AI Deployment
Pensieve plays a crucial role in simplifying the deployment of stateful large language models. By providing a streamlined framework, it allows developers to focus on model development rather than infrastructure management. This results in faster deployment times and improved overall efficiency.
Research conducted by MIT demonstrates that Pensieve can reduce deployment costs by up to 40% while maintaining high performance levels. This makes it an attractive option for businesses looking to implement AI solutions without incurring significant expenses.
Pensieve's Architecture
Core Components
Pensieve's architecture consists of several core components that work together to ensure seamless stateful model serving. These components include:
- Model Serving Layer: Handles the deployment and execution of models
- Memory Management System: Manages stateful interactions and data retention
- Optimization Engine: Enhances performance through resource allocation and caching
How It Works
The process begins with the model serving layer, which receives input data and routes it to the appropriate model. The memory management system then retrieves relevant context from previous interactions, ensuring that the model's response is context-aware. Finally, the optimization engine fine-tunes the system's performance by allocating resources efficiently.
This architecture allows Pensieve to handle high volumes of data while maintaining low latency, making it ideal for real-time applications.
Benefits of Using Pensieve
Performance Optimization
One of the primary benefits of Pensieve is its ability to optimize performance. By efficiently managing resources and leveraging advanced caching techniques, Pensieve ensures that models operate at peak efficiency. This results in faster response times and improved user satisfaction.
Scalability
Pensieve's scalable architecture enables it to handle growing workloads without compromising performance. Whether you're deploying a single model or managing a fleet of models, Pensieve can adapt to meet your needs. This scalability makes it a versatile solution for businesses of all sizes.
Challenges in Stateful Model Serving
Memory Management
One of the main challenges in stateful model serving is memory management. Stateful models require significant memory resources to store and process data, which can lead to increased costs and complexity. Pensieve addresses this challenge by implementing advanced memory management techniques that minimize resource usage while maintaining performance.
Latency Issues
Latency is another challenge in stateful model serving, particularly in real-time applications. High latency can result in slow response times, negatively impacting user experience. Pensieve mitigates this issue through its optimization engine, which reduces latency by optimizing resource allocation and caching strategies.
Use Cases of Pensieve
Chatbots and Virtual Assistants
Pensieve is particularly well-suited for chatbots and virtual assistants, where context-aware interactions are essential. By maintaining a memory of previous interactions, Pensieve enables these applications to provide more personalized and engaging experiences for users.
Customer Service Automation
In customer service automation, Pensieve's ability to handle stateful interactions ensures that users receive accurate and relevant responses. This capability can lead to improved customer satisfaction and reduced operational costs for businesses.
Comparison with Other Systems
Advantages Over Traditional Systems
Compared to traditional systems, Pensieve offers several advantages, including:
- Improved performance through advanced optimization techniques
- Enhanced scalability to accommodate growing workloads
- Reduced costs through efficient resource management
Competitive Landscape
While there are several frameworks available for stateful model serving, Pensieve stands out due to its innovative architecture and feature set. Competitors such as TensorFlow Serving and TorchServe offer similar capabilities but lack the advanced memory management and optimization features provided by Pensieve.
Optimizing Pensieve Performance
Best Practices
To maximize Pensieve's performance, consider the following best practices:
- Regularly update the model serving layer to incorporate the latest advancements
- Monitor memory usage and adjust settings as needed to optimize resource allocation
- Utilize caching strategies to reduce latency and improve response times
Troubleshooting Tips
In case of performance issues, refer to the following troubleshooting tips:
- Check for memory leaks and resolve them promptly
- Review resource allocation settings and make adjustments as necessary
- Consult the Pensieve documentation for guidance on resolving common issues
Future of Stateful LLM Serving
Emerging Trends
The future of stateful large language model serving looks promising, with emerging trends such as edge computing and quantum computing poised to further enhance capabilities. Pensieve is well-positioned to adapt to these trends, ensuring that it remains a leading solution in the field.
Potential Developments
As technology continues to evolve, we can expect to see developments in areas such as:
- Enhanced memory management techniques
- Improved optimization algorithms
- Integration with emerging AI technologies
Conclusion
In conclusion, stateful large language model serving with Pensieve represents a significant advancement in AI deployment. By addressing the challenges of memory management, scalability, and latency, Pensieve offers a robust solution for businesses and developers seeking to harness the power of stateful models. Its innovative architecture and feature set make it a standout choice in the competitive landscape of AI frameworks.
We encourage you to explore Pensieve further and consider its potential applications in your projects. For more information, feel free to leave a comment or share this article with others who may find it valuable. Together, we can continue to push the boundaries of what AI can achieve.
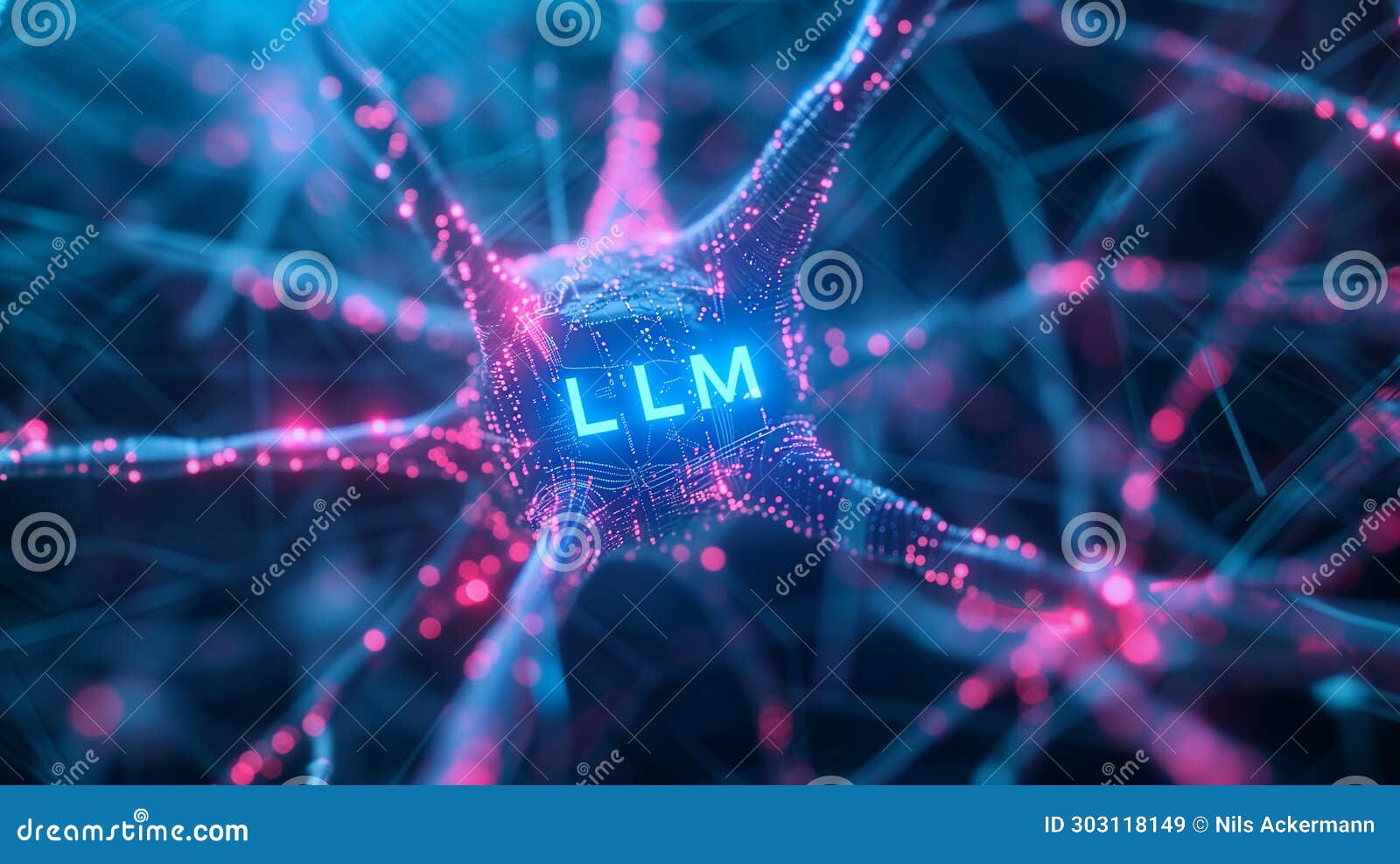
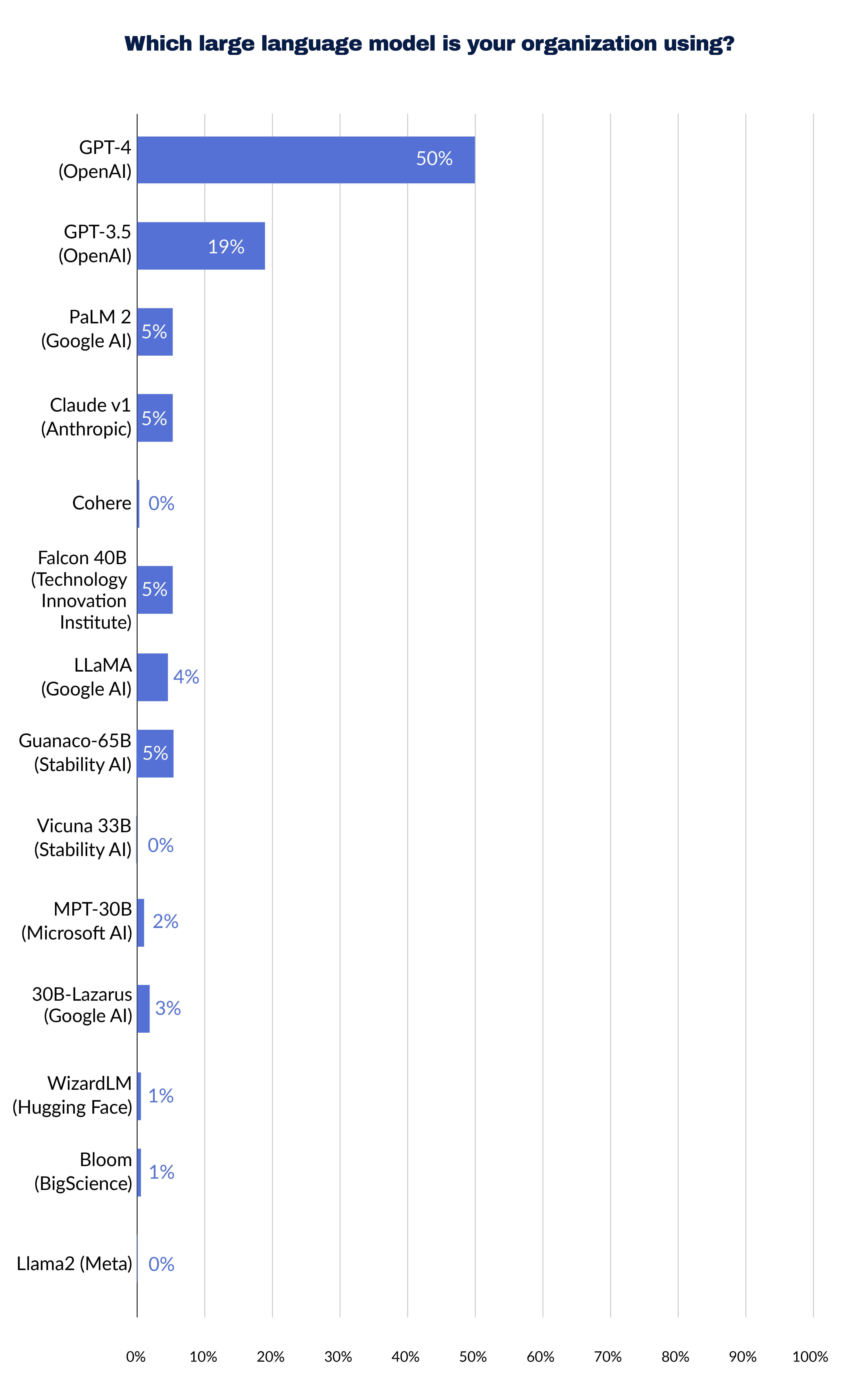
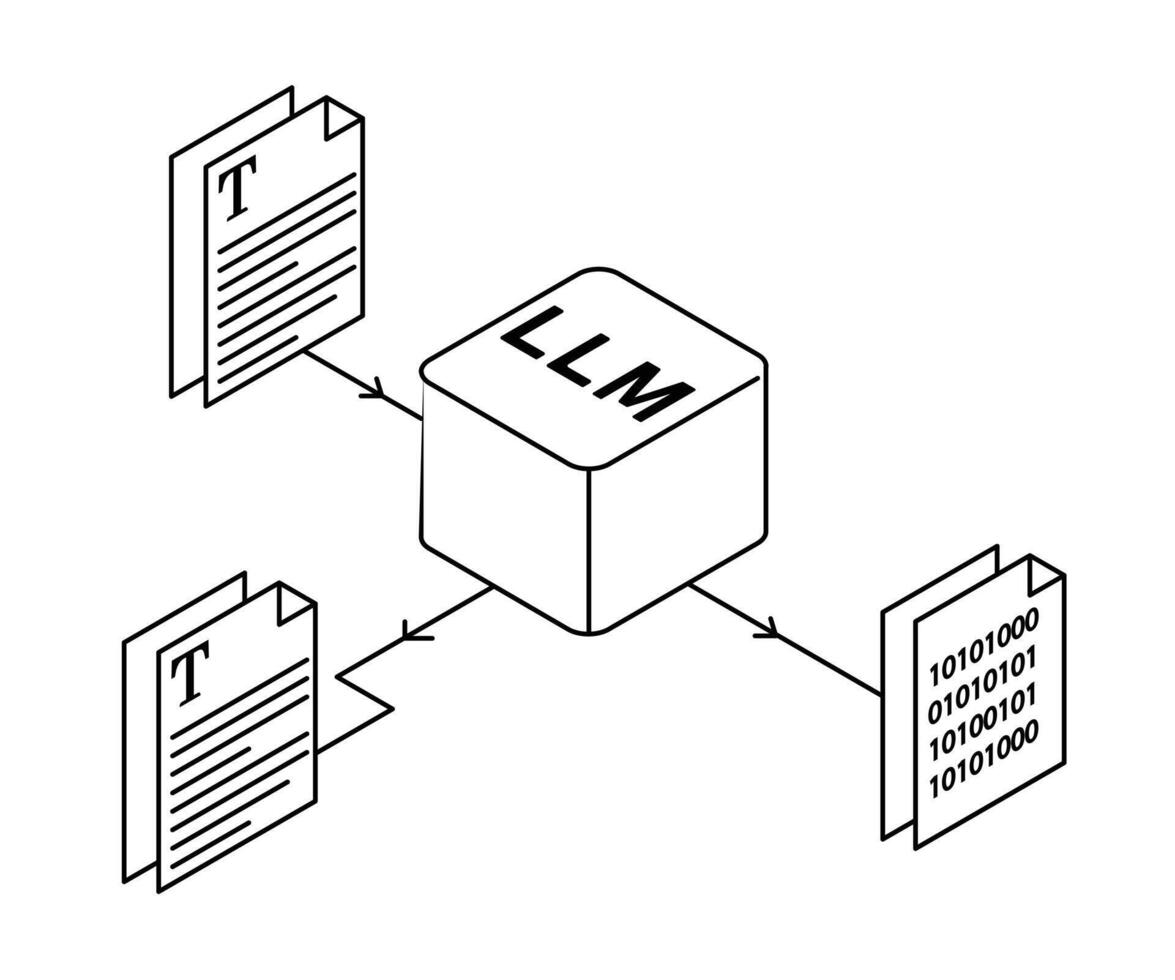